Stein-O'Brien Lab at Johns Hopkins University
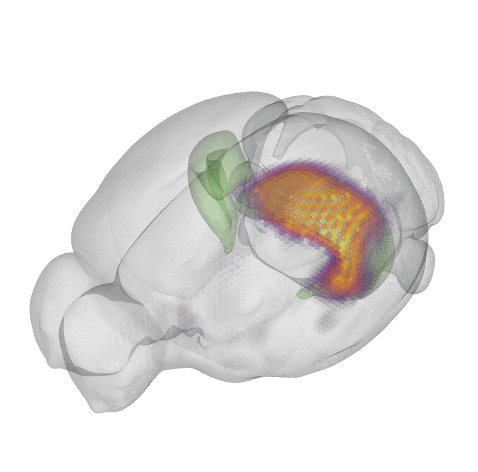
How does your genetic background manifest the phenotypic differences which make you unique?
Genetic studies have demonstrated how most traits and complex diseases are the consequence of the interactions of many genes with small effect size. However, how this manifest on a cellular level is still unclear. By studying inter and intra cellular variation, development and diseases processes can be used to uncover the functional consequence of the inherent heterogeneity encoded in an individuals genome. For example, the same mutation in C9orf72 can lead to either Amyotrophic Lateral Sclerosis (ALS) or Frontal Temporal Dementia (FTD) depending on an individual genetic background. We are currently funded by a K99/R00 from the NIH BRAIN Initiative to study this phenomena as a mechanism for developing methods to use human disease as a source of phenotypic and genotypic variation. The lab has partnered with AnswerALS in this endeavor.
How do we learn the biological processes driving a cells behavior?
Single cell gene expression profiling has demonstrated that cell classification requires more than a simple collection of marker genes. Current approaches do not account for the dynamic nature of cell states and inherent variation in cell types. This is especially true in the central nervous system (CNS) where morphological, location, and activity- based definitions of cell types fail to correlate well with single cell transcriptional profiles. Given how cell these cell extrinsic features are essential for the collective behavior and functionality of individual cells, it is critical to resolve how cell intrinsic molecular programs interact with and affect cell extrinsic physiology. A primary challenge is the need to deconvolve a single measurement of a molecular species, i.e. a single gene’s activity, in a cell into the multiple biological processes in which it is being used.Thus, my lab develops dimension reduction techniques to learn signatures of biological processes from single cell data, 2) transfer learning algorithms to assess the activity of those biological processes in other cells, molecular modalities, and species; and 3) mathematical models to predict the effects of specific perturbations of biological processes on a cell's behavior.
How can integrating across different data types and time scales inform our understanding of molecular mechanisms?
Gene expression and protein levels are often uncorrelated. The direction of effect between epigenetics and expression is still unclear. By integrating multiomic time course data, we can begin to parse out these critical regulatory relationships and better understand the molecular mechanism at play. The machine-learning subdomain of transfer learning exploits the fact that if two datasets share common latent spaces, a feature mapping between the two can identify and characterize relationships between the data defined by individual latent spaces. In this framework, one dataset is the source in which the latent space representation is learned, and another is the target that is mapped into the latent spaces learned in the source. The distribution, domain, or feature space of the source and target data may differ. Thus, transfer-learning techniques are ideally suited to assess shared latent spaces across different high thoughput molecular assays. Once the robustness of a biological process is established across systems, these approaches can also be applied to use these learned latent spaces to enable exploration of process use across data platforms, modalities, and studies. These latent spaces can also be used to parameterize mathematical models for a more mechanistic representation of the biology.
We are committed to building a laboratory and training environment that is diverse and inclusive to welcome, and collectively benefit from, unique perspectives on both science and life.
We are proud to be/have been supported by the following organizations